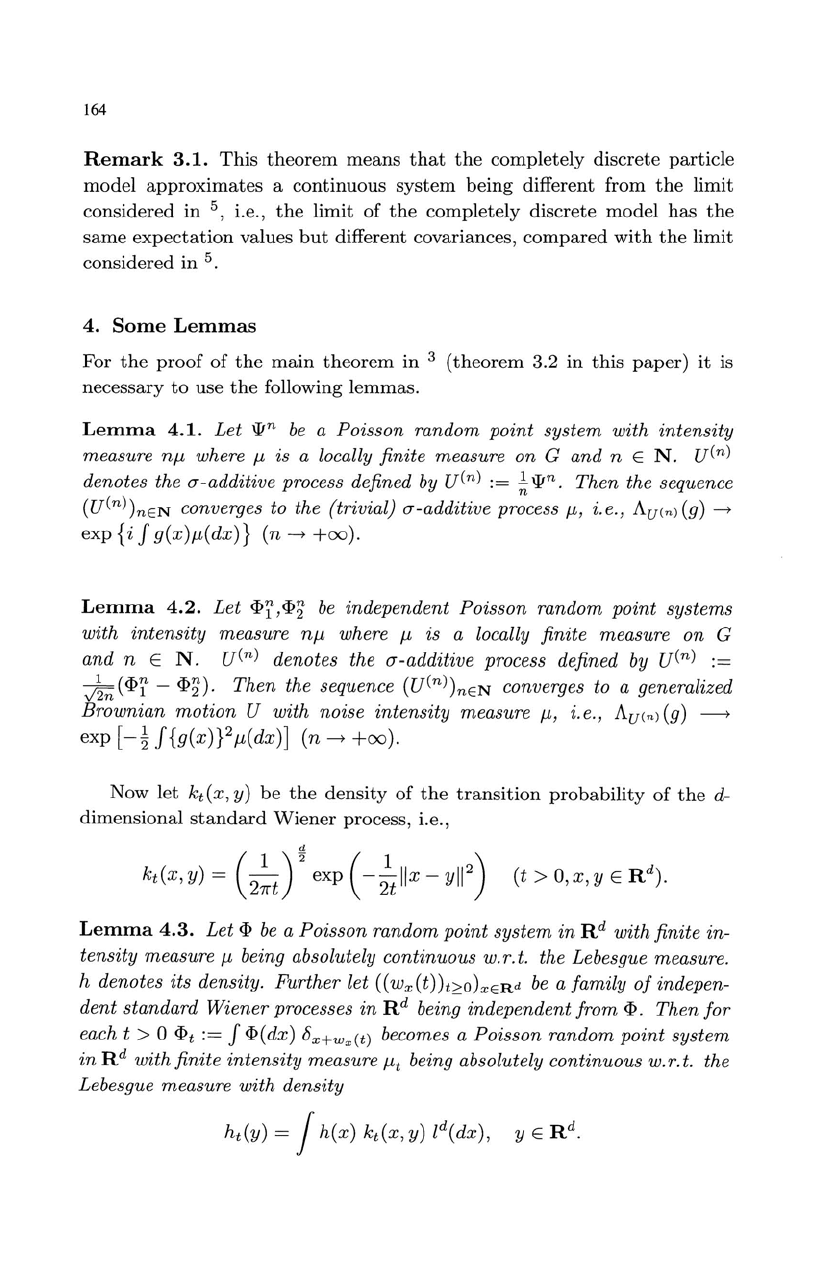
164
Remark
3.1.
This
theorem
means
that
the
completely discrete particle
model
approximates
a continuous
system
being different from
the
limit
considered
in
5,
i.e.,
the
limit
of
the
completely discrete model
has
the
same
expectation
values
but
different covariances,
compared
with
the
limit
considered in
5.
4.
Some
Lemmas
For
the
proof
of
the
main
theorem
in 3 (theorem 3.2
in
this
paper)
it
is
necessary
to
use
the
following lemmas.
Lemma
4.1.
Let
\[Tn
be
a Poisson random point
system
with
intensity
measure
nf.1
where
f.1
is a locally finite measure on G
and
n E
N.
u(n)
denotes the CT-additive process defined by
u(n)
:=
~
\[Tn.
Then
the sequence
(u(n)
)nEN
converges to the (trivial) CT-additive process
f.1,
i. e.,
AUCn)
(g)
-+
exp{ifg(x)f.1(dx)}
(n-++oo).
Lemma
4.2.
Let
<pr,<p~
be
independent Poisson random
point
systems
with
intensity
measure
nf.1
where
f.1
is a locally finite measure on G
and n
E
N.
u(n)
denotes the CT-additive process defined by
u(n)
:=
vk(<p
1
-
<p~).
Then
the sequence
(u(n))nEN
converges to a generalized
Brownian
motion
U with noise
intensity
measure
f.1,
i.e.,
AUCn)
(g)
--+
exp
[-~
f{g(X)}2f.1(dx)]
(n
-+
+00).
Now let
kt(x
, y)
be
the
density
of
the
transition
probability
of
the
d-
dimensional
standard
Wiener
process, i.e.,
d
kt(x,y)
=
(_1_)"2
exp
(-~llx
_
Y112)
(t>
Q,x,y
E R
d
).
27rt
2t
Lemma
4.3.
Let
<P
be
a
Poisson
random point
system
in
R d with finite in-
tensity
measure
f.1
being absolutely continuous
w.
r.
t.
the Lebesgue measure.
h denotes its density. Further let
((w
x
(t))t20)XERd
be
a family
of
indepen-
dent standard
Wiener
processes
in
R d being independent
from
<P.
Then
for
each t > Q
<P
t
:=
f
<P(dx)
Dx+wx(t)
becomes a Poisson random point
system
in
R d with finite
intensity
measure
f.1t
being absolutely continuous
w.
r.
t.
the
Lebesgue measure with density