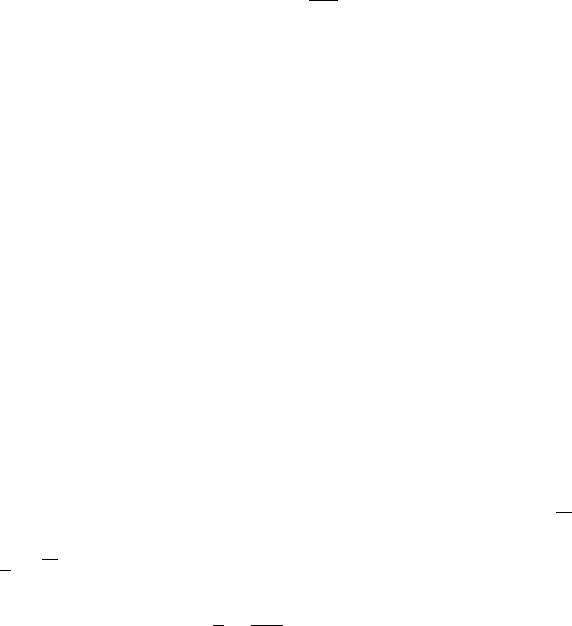
tends to zero. In other words, random variable Y/n approaches the true mean
with probability 1.
Answer: to proceed with the proof of Equation (4.44), we first note that, if
is the variance of each X
j
, it follows from Equation (4.41) that
According to the Chebyshev inequality, given by Expression (4.17), for every
0, we have
For the left-hand side is less than
which tends to zero as
n . This establishes the proof.
Note that this proof requires the existence of
2
. This is not necessary but
more work is required without this restriction.
Among many of its uses, statistical sampling is an example in which the law of
large numbers plays an important role. Suppose that in a group of m families
there are m
j
number of families with exactly j children ( j 0, 1, . . . , and
m
0
m
1
... m). For a family chosen at random, the number of children is
a random variable that assumes the value r with probability p
r
m
r
/m. A sample
of n families among this group represents n observed independent random
variables X
1
,...,X
n
, with the same distribution. The quantity (X
1
X
n
)/n
is the sample average, and the law of large numbers then states that, for
sufficientlylargesamples,thesampleaverageislikelytobecloseto
the mean of the population.
Ex ample 4. 13. The random variable Y/n in Example 4.12 is also called the
sample mean associated with random variables X
1
,...,X
n
and is denoted by X.
In Example 4.12, if the coefficient of variation for each X
i
is v, the coefficient of
variation v
X
of X is easily derived from Equations (4.38) and (4.41) to be
Equation (4.45) is the basis for the law of by Schr ödinger, which states that
the laws of physics are accurate within a probable relative error of the order of
where n is the number of molecules that cooperate in a physical process.
Basically, what Equation (4.45) suggests is that, if the action of each molecule
Expectations and Moments 97
"
2
2
Y
n
2
:
k >
PjY nmjk
n
2
k
2
:
k "n,
2
/("
2
n),
!1
m
X
r0
rp
r
X
r0
rm
r
=m;
v
X
v
n
1=2
4:45
n
p
n
1/2
,