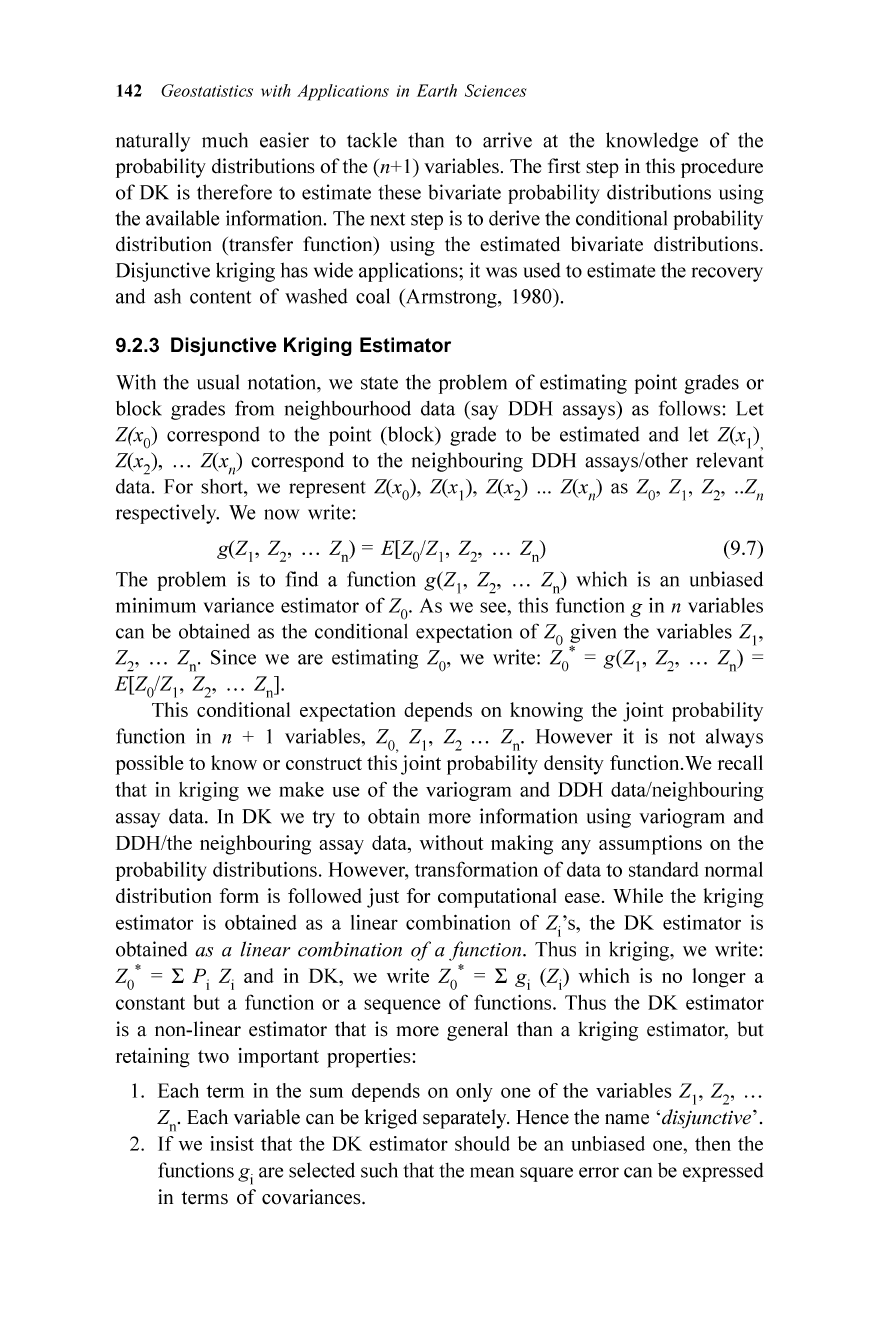
142 Geostatistics with Applications in Earth Sciences
naturally much easier to tackle than to arrive at the knowledge
of
the
probability distributions
of
the (n
+l)
variables. The first step in this procedure
of
OK is therefore to estimate these bivariate probability distributions using
the available information . The next step is to derive the conditional probability
distribution (transfer function) using the estimated bivariate distributions.
Disjunctive kriging has wide applications; it was used to estimate the recovery
and ash content
of
washed coal (Armstrong, 1980).
9.2.3
Disjunctive Kriging Estimator
With the usual notation, we state the problem
of
estimating point grades or
block grades from neighbourhood data (say DOH assays) as follows: Let
Z(x
o)
correspond to the point (block) grade to be estimated and let Z(x
l
)
Z(x
2)
, ... Z(x
n)
correspond to the neighbouring DOH assays /other relevant
data. For short, we represent
Z(x
o)
' Z(x
l
) ,
Z(x
2)
... Z(x,) as z; ZI' Z2' "Zn
respectively. We now write:
g(ZI'
Z2
' ... Zn) =
E[Z
alZI'
Z2
' .. . Zn) (9.7)
The problem is to find a function
g(ZI'
Z2
' ... Zn) which is an unbiased
minimum variance estimator
of
ZO
oAs we see, this function g in n variables
can be obtained as the conditional expectation
of
Zo
given the variables
ZI'
Z2
' .. . Zn' Since we are estimating Zo' we write: Zo' =
g(ZI'
Z2
' .. . Zn) =
E[Z
alZI' Z2' ... Zn]'
This conditional expectation depends on knowing the joint probability
function in n
+ I variables,
Zo
, ZI' Z2 ... Zn' However it is not always
possible to know or construct this
joint
probability density function. We recall
that in kriging we make use
of
the variogram and DOH data/neighbouring
assay data. In OK we try to obtain more information using variogram and
DOH/the neighbouring assay data, without making any assumptions on the
probability distributions. However, transformation
of
data to standard normal
distribution form is followed
just
for computational ease. While the kriging
estimator is obtained as a linear combination
of
Zj'S, the OK estimator is
obtained
as a linear combination
of
a function. Thus in kriging , we write:
Zo
* = L P, Zj and in OK, we write Zo* = L gj
(Z)
which is no longer a
constant but a function or a sequence
of
functions. Thus the OK estimator
is a non-linear estimator that is more general than a kriging estimator, but
retaining two important properties:
I. Each term in the sum depends on only one
of
the variables
ZI'
Z2' ...
Zn'
Each variable can be kriged separately. Hence the name 'disj unctive' .
2. If we insist that the OK estimator should be an unbiased one, then the
functions
gj are selected such that the mean square error can be expressed
in terms
of
covariances.