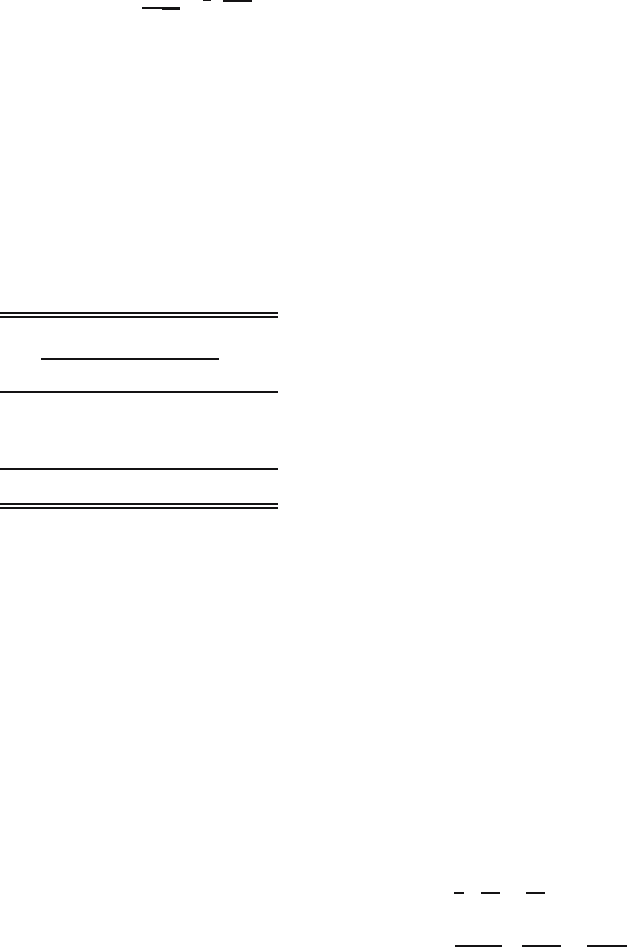
438 C Answers to selected exercises
9.19 b Use that
1
σ
√
2π
e
−
1
2
y−µ
σ
2
is
the probability density function of an
N(µ, σ
2
) distributed random variable.
9.19 c N (0, 1/36).
10.1 a Cov(X, Y )=0.142. Positively
correlated.
10.1 b ρ (X, Y )=0.0503.
10.2 a E[XY ]=0.
10.2 b Cov(X, Y )=0.
10.2 c Var(X + Y )=4/3.
10.2 d Var(X − Y )=4/3.
10.5 a
a
b 012
0 8/72 6/72 10/72 1/3
1 12/72 9/72 15/72 1/2
2 4/72 3/72 5/72 1/6
1/3 1/4 5/12 1
10.5 b E[X]=13/12, E [Y ]=5/6, and
Cov(X, Y )=0.
10.5 c Yes.
10.6 a E[X]=E[Y ]=0and
Cov(X, Y )=0.
10.6 b E[X]=E[Y ]=c;E[XY ]=c
2
.
10.6 c No.
10.7 a Cov(X, Y )=−1/8.
10.7 b ρ(X, Y )=−1/2.
10.7 c For ε equal to 1/4, 0 or −1/4.
10.9 a P(X
i
=1)=(1−0.001)
40
=0.96
and P(X
i
= 41) = 0.04.
10.9 b E[X
i
]=2.6and
E[X
1
+ ···+ X
25
] = 65.
10.10 a E[X] = 109/50,
E[Y ] = 157/100, and E [X + Y ]=15/4.
10.10 b E
X
2
= 1287/250,
E
Y
2
= 318/125, and
E[X + Y ] = 3633/250.
10.10 c Var(X) = 989/2500,
Var(Y ) = 791/10 000, and
Var(X + Y ) = 4747/10 000.
10.14 a Use the alternative expression
for the covariance.
10.14 b Use the alternative expression
for the covariance.
10.14 c Combine parts a and b.
10.16 a Var(X)+Cov(X, Y ).
10.16 b Anything can happen.
10.16 c X and X +Y are positively cor-
related.
10.18 Solve 0 = N(N −1)(N +1)/12 +
N(N − 1)Cov(X
1
,X
2
).
11.1 a Check that for k between 2 and 6,
the summation runs over =1,...,k−1,
whereas for k between 7 and 12 it runs
over = k − 6,...,12.
11.1 b Check that for 2 ≤ k ≤ N,the
summation runs over =1,...,k − 1,
whereas for k between N +1 and 2N it
runs over = k − N,...,2N.
11.2 a Check that the summation runs
over =0, 1,...,k.
11.2 b Use that λ
k−
µ
/(λ+µ)
k
is equal
to p
1 − p
k−
,withp = µ/(λ + µ).
11.4 a E[Z]=−3andVar(Z) = 81.
11.4 b Z has an N(−3, 81) distribution.
11.4 c P(Z ≤ 6) = 0.8413.
11.5 Check that for 0 ≤ z<1, the in-
tegral runs over 0 ≤ y ≤ z,whereasfor
1 ≤ z ≤ 2, it runs over z − 1 ≤ y ≤ 1.
11.6 Check that the integral runs over
0 ≤ y ≤ z.
11.7 Recall that a Gam (k, λ) random
variable can be represented as the sum of
k independent Exp (λ) random variables.
11.9 a f
Z
(z)=
3
2
1
z
2
−
1
z
4
,forz ≥ 1.
11.9 b f
Z
(z)=
αβ
β − α
1
z
β+1
−
1
z
α+1
,
for z ≥ 1.
12.1 e 1: no, 2: no, 3: okay, 4: okay, 5:
okay.
12.5 a 0.00049.